ReAct: Teaching AI Models to Think & Do Like Us
- lekhakAI
- Jun 13, 2024
- 4 min read
Large Language Models (LLMs) are all the rage these days. They can write poems, translate languages, and even have a decent conversation. But how good are they at combining thinking and acting to solve complex problems?
That's where ReAct comes in.
Think of ReAct as a personal trainer for LLMs, helping them flex their "reasoning" and "acting" muscles. Instead of treating these skills as separate entities, ReAct encourages LLMs to use them in tandem, creating a powerful synergy.
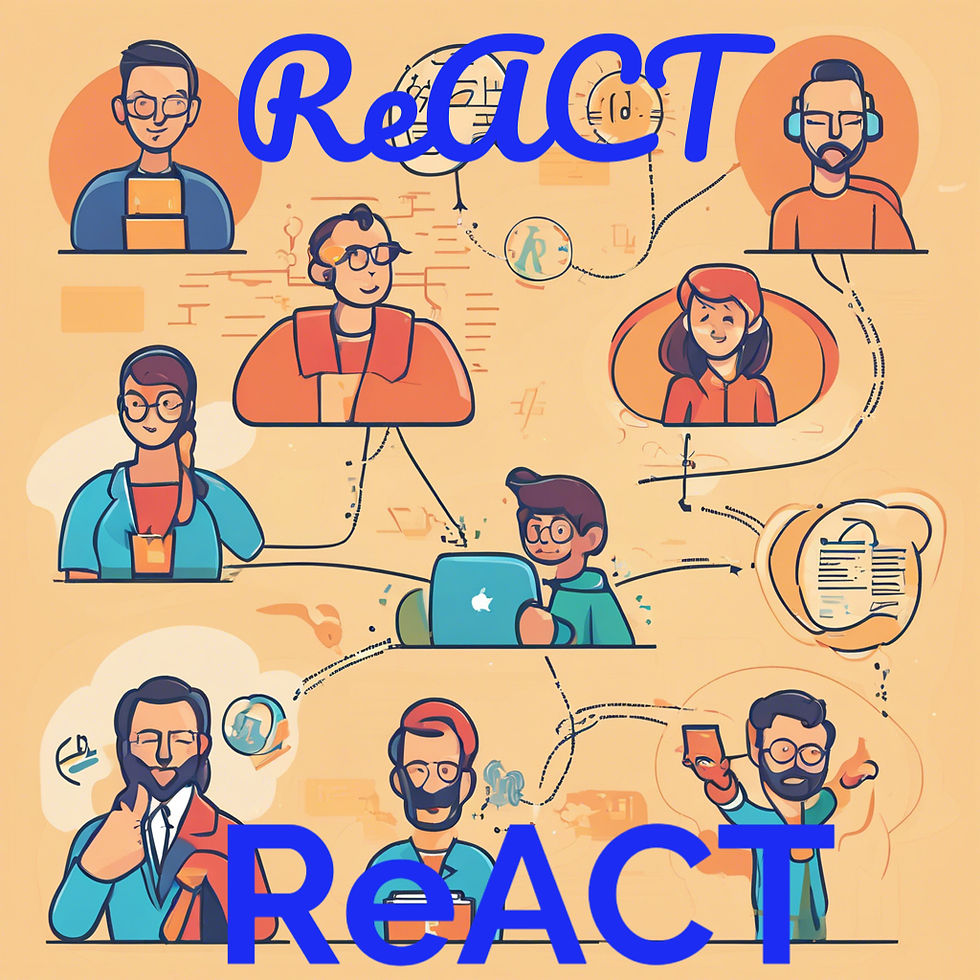
Let's break it down:
Imagine you're trying to bake a cake. You wouldn't just blindly throw ingredients together, right? You'd follow a recipe, think about each step, and adjust your actions based on the outcome.
That's what ReAct aims to do with LLMs.
Reasoning: This is like the LLM's internal monologue, where it breaks down a task into smaller steps, just like you would with your cake recipe. It's all about planning and understanding the "why" behind each action.
Acting: This is where the LLM interacts with the real (or digital) world. It could be searching for information online, accessing a database, or even controlling a robot arm to frost that cake.
Synergy in Action:
ReAct's magic lies in its ability to make reasoning and acting work hand-in-hand.
Reasoning guides to action: The LLM's internal monologue helps it choose the most effective actions. For example, if the recipe calls for "softened" butter and the LLM only has cold butter, its reasoning will guide it to search for ways to soften it.
Action informs reasoning: The LLM learns from its actions and updates its plan accordingly. Let's say the LLM tries microwaving the butter to soften it (don't try this at home!), but it melts. This experience will inform its reasoning, leading it to explore other options.
Why is ReAct a Big Deal?
ReAct isn't just another fancy acronym in the AI world. It represents a significant leap forward in LLM capabilities. Here's why:
Reduced Hallucination: LLMs are notorious for making things up, especially when they lack real-world context. ReAct helps ground their reasoning by allowing them to access external information sources, like a Wikipedia search for that butter-softening dilemma.
Improved Interpretability: Have you ever wondered what goes on inside an LLM's "brain"? ReAct makes the decision-making process more transparent by providing insights into the LLM's reasoning. It's like having access to the LLM's thought process, step-by-step.
Enhanced Trustworthiness: By making LLMs more transparent and less prone to errors, ReAct paves the way for their use in more sensitive and critical applications. Imagine an LLM assisting doctors with diagnoses or helping lawyers with legal research – ReAct brings us closer to that reality.
Real-World Results:
ReAct has been put to the test in various tasks, and the results are impressive.
Question Answering & Fact Verification: ReAct outshines traditional LLMs by using its reasoning to identify relevant information and its acting to verify facts against external sources.
Interactive Decision Making: ReAct excels in complex scenarios like navigating a virtual world or making purchasing decisions in an online store, demonstrating its ability to learn and adapt to dynamic environments.
The Future of ReAct:
ReAct is still a young technology, but its potential is immense. As researchers continue to refine and develop this approach, we can expect to see LLMs that are:
More capable: Solving even more complex tasks that require a high level of reasoning and interaction.
More versatile: Adapting to a wider range of domains and applications.
More human-like: Thinking and acting in ways that are increasingly aligned with human cognition.
ReAct represents a significant step towards creating LLMs that are not just intelligent but also capable of understanding and interacting with the world around them. It's an exciting time to be following the development of this groundbreaking technology!
FAQs
1. How is ReAct different from traditional language models?
Traditional LLMs primarily excel at language understanding and generation. They might generate impressive text, but their ability to reason logically and interact with the world is limited. ReAct, on the other hand, explicitly trains LLMs to combine reasoning and acting. It's like teaching an LLM not just to read about baking a cake but also to understand the steps involved and potentially even execute them.
2. What are some potential applications of ReAct in the real world?
The possibilities are vast! ReAct could revolutionize:
Customer service: Imagine AI assistants who can understand complex requests, navigate knowledge bases, and provide personalized solutions, all while explaining their reasoning.
Education: ReAct could power interactive learning platforms where students receive personalized guidance and feedback based on their learning styles and needs.
Healthcare: From assisting doctors with diagnoses to managing patient records and even controlling robotic surgical tools, ReAct could transform healthcare delivery.
3. What are the limitations of ReAct?
While promising, ReAct is still under development. Some limitations include:
Data dependency: ReAct relies heavily on large datasets for training, which can be a bottleneck for specific domains or tasks.
Bias and fairness: Like all AI models, ReAct can inherit biases present in the training data, leading to potentially unfair or discriminatory outcomes.
Explainability: While ReAct offers more transparency than traditional LLMs, fully understanding and interpreting the reasoning process of complex models remains a challenge.
4. Is ReAct accessible to everyone?
Currently, ReAct requires significant computational resources and technical expertise to implement. However, as with many AI advancements, we can expect to see more accessible tools and platforms emerge in the future, making ReAct's capabilities available to a wider audience.
5. What's next for ReAct?
The future of ReAct is full of exciting possibilities! Researchers are continuously working on:
Improving efficiency: Making ReAct less computationally expensive and easier to train.
Addressing bias: Developing techniques to mitigate bias and ensure fairness in ReAct's decision-making.
Expanding capabilities: Exploring new applications and tasks where ReAct's unique strengths can be leveraged.
As ReAct continues to evolve, we can expect to see even more innovative applications that push the boundaries of what's possible with AI.
Comments